A Distributed Platform’s Powerful Ability to Create Predictive AI Models and Accelerate Precision Oncology

KEY INSIGHTS
- Artificial intelligence (AI), when combined with real-world data access, can accelerate the full potential of precision oncology, as well as precision medicine more broadly.
- Distributed AI models provide data insights for diverse patient populations and practice settings to advance innovative cancer care solutions.
- A rigorous and transparent process is necessary. From the onset, all factors that might influence the development of a successful predictive AI-based biomarker must be taken into consideration in order to maximize the project’s chances of success.
- Industry players can access new AI resources to reinforce clinical-trial predictive enrichment strategies and inform care pathway decisions with the benefit of tailoring approaches to patient care.
- An included real-world example illustrates how to apply a deep-learning model to predict clinical outcomes in patients with advanced non-small cell lung cancer who were treated with immune checkpoint inhibitors (ICI).
AI-Driven Insights and NGS Testing Are Accelerating Precision Medicine
Precision medicine aims to offer a patient, or a subgroup of patients, disease treatment and prevention that is optimally efficient and/or beneficial for them. This is based on their genetic predisposition, their environment, and their lifestyle.
While precision medicine is not a new approach to disease treatment and prevention, progress in biology and disease knowledge — combined with improved data-collection technologies — has led to increased improvements. This is because AI has the power to extract the relevant information from these vast quantities of data and transform it into actionable knowledge — which helps predict disease and targeted therapy.
Avitia combines advanced AI, local NGS testing, and secure data workflows to deliver actionable insights quickly. This includes empowering local labs to rapidly and accurately identify cancer mutations, as well as provide up-to-date treatment insights. This network intelligence drives precision medicine advancements while benefiting from collective insights across diverse patient cohorts, jurisdictions, and therapeutic indications.
AI-based Predictive Biomarkers: A Real-World Application
Predictive biomarkers are used to identify individuals who are more likely than similar individuals without the biomarker to experience a favorable or unfavorable effect from exposure to a medical product or an environmental agent.
As such, predictive biomarkers can be fruitfully applied in clinical-trial settings to improve enrichment strategies. A predictive enrichment strategy uses predictive biomarkers to more rapidly and efficiently identify patients who are more likely to respond to a treatment, as well as to determine cohort stratification. Predictive biomarkers can also assist in informing patient-care decisions, for instance, in identifying a treatment that is more likely to benefit the patient.
An example of predictive biomarkers is that of immune checkpoint inhibitor (ICI) drugs, which are novel therapeutic agents used notably in treating patients with non-small cell lung cancer (NSCLC). Capturing the dynamic nature of interactions between a patient's immune system and a tumor generates volumes of diverse data that are difficult to interpret in any practical way. Using AI in the discovery process is a promising approach to analyzing this complex data — especially from collective insights across diverse patient cohorts. This allows clinicians to tailor treatment plans and improve patient outcomes by putting the patients on an effective treatment faster.
The potential of this application of AI-based biomarkers is well illustrated in this project led by Avitia’s Dr. Kam Kafi: Deep learning model to predict clinical outcomes in patients with advanced non-small cell lung cancer treated with immune checkpoint inhibitors.
The AI-based Biomarker Discovery Process
At Avitia, our AI-driven assays are supported by robust, sensitive, and high-throughput genomic data analytics for accurate cancer mutation identification. Our system uses rigorously validated AI/Machine learning-based analytical and clinical reporting pipelines.
These distributed insights drive the discovery process of an AI-based biomarker — from framing the clinical/business needs to the biomarker discovery phase and up to the productization strategy.
Our sequential, multidimensional process is driven by a multidisciplinary team of professionals as well as an extensive network of collaborators through our distributed AI platform.
Our process is designed to handle the massive, multimodal data that exists in healthcare — which must be accessed, mined, structured, and analyzed — while simultaneously protecting patient privacy.
In order to do so, we leverage our cutting-edge machine learning and medical expertise, privacy-by-design approach, and robust validation phase. The entire sequence is supported by industrial project management standards.
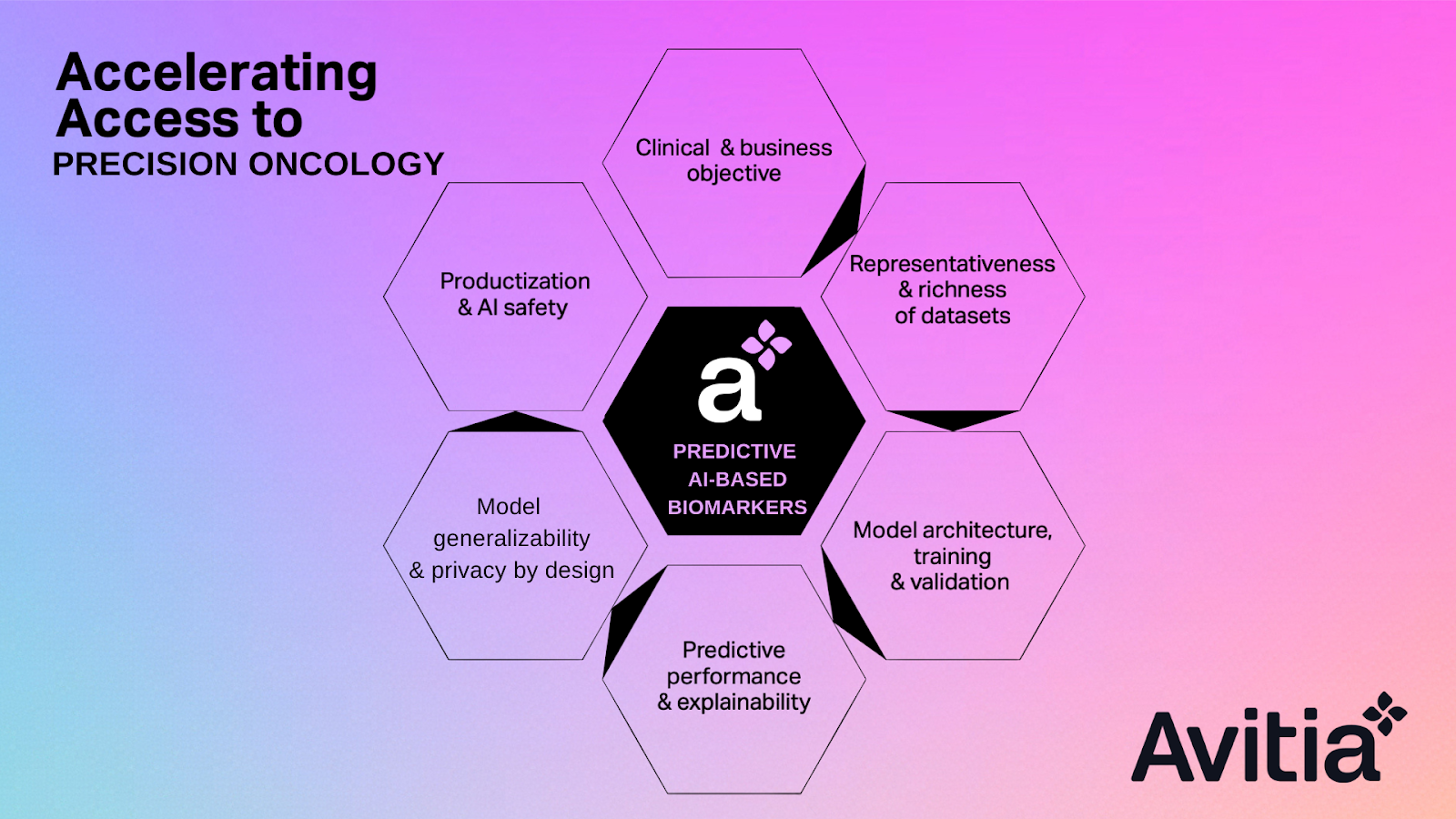
All factors that could influence the development of a successful predictive AI-based biomarker are taken into consideration in order to maximize the project’s chances of success.
- Clinical need and business value: By first considering the current care pathway approach and the need to optimize patient management and/or to personalize the therapeutic strategy, we frame the clinical objective and the benefits expected by using AI in the discovery process.
- Intent of use: The biomarker development plan is adjusted according to the context of the AI-based biomarker’s use, for instance, in support of a clinical trial, within a real-world-evidence study, to ensure the appropriate usage of a marketed drug or a clinical decision tool.
- Multiple data sources: It could be real-world data accessed via our collaborative partnerships, clinical trials data, patient-reported outcomes, registries, etc. This has an impact on:
- The level of data curation and preparation needed to generate an AI-ready dataset that meets a regulatory-grade data status,
- The data linkage,
- The infrastructure and the access rules that should be considered (on-premise or on the cloud),
- The data governance and security issues, and the compliance to the international standards (HIPAA, PIPEDA, GDPR).
- Data types and quality. Data can be unstructured or structured and can take on many forms — text, images, genomics, among others. That, in combination with the size, representativeness, and richness of the datasets, impacts data preprocessing and the scope of the biomarker design process.
- Model development. A multitude of AI approaches can be investigated and used, including self-evolving machine learning, deep radiomics, multimodal learning, transfer learning, or Natural Language Processing (NLP). The selected AI solution is tailored to the biomarker candidate targeted and the data types. Models are first evaluated on their predictive performance, interpretability, possible bias, reproducibility, and generalizability. The statistical plan and methodology are a central point of discussion for the AI model architecture, training, and validation. Candidate biomarkers undergo further fit-for-purpose validation and/or are evaluated for clinical benefit.
- Privacy by design. The generalizability and validation of the learnings on various datasets without moving the data from their location is facilitated by our advanced federated learning approach, which allows us to preserve data privacy while scaling learnings. If appropriate, privacy can also be reinforced by using homomorphic encryption.
- Regulatory environment. If the biomarker candidate is destined to become a clinical-grade product, for instance, a Software as a Medical Device, a regulatory strategy is prepared and implemented from the proof of concept stage.
The biomarker discovery process is also built on fundamental principles such as traceability, security, and transparency. These factors facilitate AI adoption by clinicians, industry, and regulated bodies — ensuring the full potential of precision medicine.
This biomarker discovery process is designed to accelerate the discovery and transformation of promising AI-based candidates into robust AI-based biomarkers that can be deployed in clinical settings, where they can accomplish our ultimate goal of improving patient outcomes.
Gardez une longueur d'avance sur le cancer dès aujourd'hui
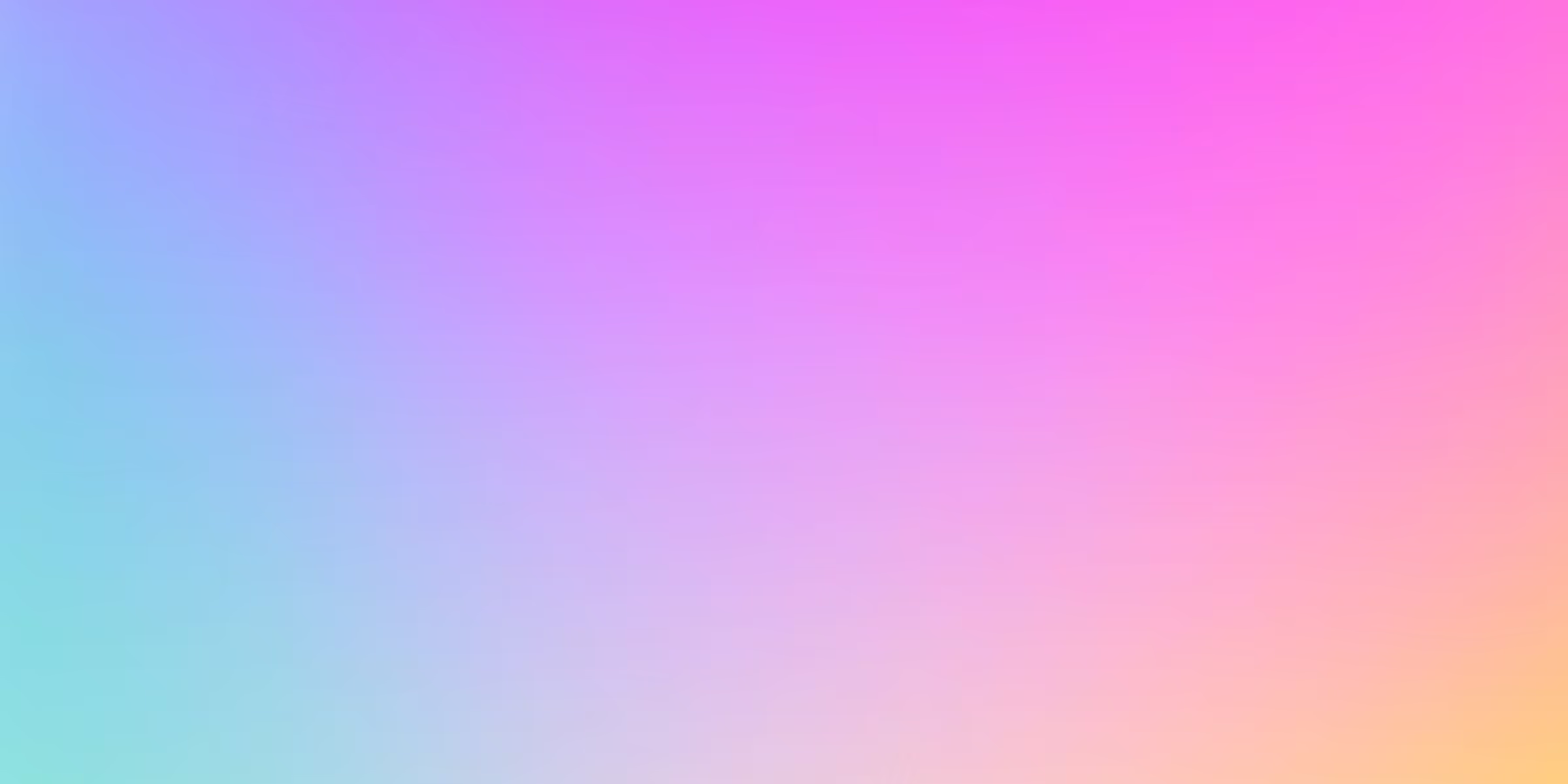